Trigonometry/For Enthusiasts/Delaunay triangulation
A Delaunay triangulation is a particular way of joining a set of points to make a triangular mesh. Delaunay triangulations tend to avoid skinny triangles. The triangulation was invented by Boris Delaunay in 1934[1].
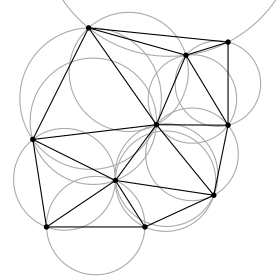
Applications
editFile:Bush-Arnie-morph.jpg | |
Morph of George W. Bush to Arnold Schwarzenegger | Finite Element Analysis solution of a fluid flow problem |
- For morphing images the Delaunay triangulation provides a 'good' way to create a triangular mesh from points that are going to be moved. Each triangle can be distorted in a simple way, leading to a complex 'morphing' distortion of the overall image. In the example of morphing shown, the triangular shapes are distorted from one image to the next, so for example the hair in the first image is distorted to fit the hair in the second. At the same time as that happens, the colours 'cross fade' from one colour to another so the grey cross fades to brown.
Sierra Nevada terrain map | 2D triangular mesh | 2D solution calculated from mesh |
- For modelling terrain or other objects given a set of sample points, the Delaunay triangulation gives a nice set of triangles to use as polygons in the model. In particular, the Delaunay triangulation avoids narrow triangles (as they have large circumcircles compared to their area).
- Delaunay triangulations are used in many other applications where a shape has to be divided up into triangles. Analysis of stresses and strains in structures is often done using a triangular mesh. In the analysis shown above, more points are put in the area of most interest to get a finer more detailed analysis in that area. That's also what we do when morphing images - we put more points where we want most control over the fine details of the morphing. If you want to change a frown into a smile, put more points around the mouth so you can change the shape more easily.
Formal Definition
editFor a set P of points in the plane the Delaunay triangulation is a triangulation DT(P) such that no point in P is inside the circumcircle of any triangle in DT(P). Delaunay triangulations maximize the minimum angle of all the angles of the triangles in the triangulation; they tend to avoid skinny triangles.
For a set of points on the same line there is no Delaunay triangulation (in fact, the notion of triangulation is undefined for this case). For four points on the same circle (e.g., the vertices of a rectangle) the Delaunay triangulation is not unique: the two possible triangulations that split the quadrangle into two triangles satisfy the "Delaunay condition", i.e., the requirement that the circumcircles of all triangles have empty interiors.
Relationship with the Voronoi diagram
editThe Delaunay triangulation of a discrete point set P in general position corresponds to the dual graph of the Voronoi tessellation for P. Special cases include the existence of three points on a line and four points on circle.
-
The Delaunay triangulation with all the circumcircles and their centers (in red).
-
Connecting the centers of the circumcircles produces the Voronoi diagram (in red).
Properties
editLet n be the number of points (vertices).
- In the plane, each vertex has on average six surrounding triangles.
- In the plane, the Delaunay triangulation maximizes the minimum angle. Compared to any other triangulation of the points, the smallest angle in the Delaunay triangulation is at least as large as the smallest angle in any other. However, the Delaunay triangulation does not necessarily minimize the maximum angle.
- A circle circumscribing any Delaunay triangle does not contain any other vertices of the triangulation in its interior.
- If a circle passing through two of the vertices doesn't contain any other of them in its interior, then the segment connecting the two points is an edge of a Delaunay triangulation of the given points.
- The closest neighbor b to any point p is an edge bp in the Delaunay triangulation. The Nearest neighbor graph is a subgraph of the Delaunay triangulation as is the minimum spanning tree.
Convex Hull
edit- The union of all triangles in the triangulation is the convex hull of the points.
- In the plane, if there are b vertices on the convex hull, then any triangulation of the points has at most 2n − 2 − b triangles, plus one exterior face (see Euler characteristic).
from here on needs a lot more editing |
Visual Delaunay definition: Flipping
editFrom the above properties an important feature arises: Looking at two triangles ABD and BCD with the common edge BD (see figures), if the sum of the angles α and γ is less than or equal to 180°, the triangles meet the Delaunay condition.
This is an important property because it allows the use of a flipping technique. If two triangles do not meet the Delaunay condition, switching the common edge BD for the common edge AC produces two triangles that do meet the Delaunay condition:
-
This triangulation does not meet the Delaunay condition (the sum of α and γ is bigger than 180°).
-
This triangulation does not meet the Delaunay condition (the circumcircles contain more than 3 points).
-
Flipping the common edge produces a Delaunay triangulation for the four points.
Algorithms
editMany algorithms for computing Delaunay triangulations rely on fast operations for detecting when a point is within a triangle's circumcircle and an efficient data structure for storing triangles and edges. In two dimensions, one way to detect if point D lies in the circumcircle of A, B, C is to evaluate the determinant:[2]
Assuming A, B and C to lie counter-clockwise, this is positive if and only if D lies in the circumcircle.
Flip algorithms
editAs mentioned above, if a triangle is non-Delaunay, we can flip one of its edges. This leads to a straightforward algorithm: construct any triangulation of the points, and then flip edges until no triangle is non-Delaunay. Unfortunately, this can take O(n2) edge flips, and does not extend to three dimensions or higher[3].
Incremental
editThe most straightforward way of efficiently computing the Delaunay triangulation is to repeatedly add one vertex at a time, retriangulating the affected parts of the graph. When a vertex v is added, we split in three the triangle that contains v, then we apply the flip algorithm. Done naively, this will take O(n) time: we search through all the triangles to find the one that contains v, then we potentially flip away every triangle. Then the overall runtime is O(n2).
If we insert vertices in random order, it turns out (by a somewhat intricate proof) that each insertion will flip, on average, only O(1) triangles – although sometimes it will flip many more[4]. This still leaves the point location time to improve. We can store the history of the splits and flips performed: each triangle stores a pointer to the two or three triangles that replaced it. To find the triangle that contains v, we start at a root triangle, and follow the pointer that points to a triangle that contains v, until we find a triangle that has not yet been replaced. On average, this will also take O(log n) time. Over all vertices, then, this takes O(n log n) time[3]. While the technique extends to higher dimension (as proved by Edelsbrunner and Shah[5]), the runtime can be exponential in the dimension even if the final Delaunay triangulation is small.
The Bowyer–Watson algorithm provides an another approach for incremental construction. It gives an alternative to edge flipping for computing the Delaunay triangles containing a newly inserted vertex.
Divide and conquer
editA divide and conquer algorithm for triangulations in two dimensions is due to Lee and Schachter which was improved by Guibas and Stolfi[6] and later by Dwyer. In this algorithm, one recursively draws a line to split the vertices into two sets. The Delaunay triangulation is computed for each set, and then the two sets are merged along the splitting line. Using some clever tricks, the merge operation can be done in time O(n), so the total running time is O(n log n).[7]
For certain types of point sets, such as a uniform random distribution, by intelligently picking the splitting lines the expected time can be reduced to O(n log log n) while still maintaining worst-case performance.
A divide and conquer paradigm to performing a triangulation in d dimensions is presented in "DeWall: A fast divide and conquer Delaunay triangulation algorithm in Ed" by P. Cignoni, C. Montani, R. Scopigno.[8]
Divide and conquer has been shown to be the fastest DT generation technique.[9][10]
Sweepline
editFortune's Algorithm uses a sweepline technique to achieve O(n log n) runtime in the planar case.
Sweephull
editSweephull[11] is a fast hybrid technique for 2D Delaunay triangulation that uses a radially propagating sweep-hull (sequentially created from the radially sorted set of 2D points, giving a non-overlapping triangulation), paired with a final iterative triangle flipping step. Empirical result indicate the algorithm runs in approximately half the time of Qhull. GPL code is available from [12]
References
edit- ↑ B. Delaunay: Sur la sphère vide, Izvestia Akademii Nauk SSSR, Otdelenie Matematicheskikh i Estestvennykh Nauk, 7:793–800, 1934
- ↑ Guibas, Lenoidas (1985-04-01). "Primitives for the manipulation of general subdivisions and the computation of Voronoi". ACM. p. 107. Retrieved 2009-08-01.
{{cite web}}
: Unknown parameter|coauthors=
ignored (|author=
suggested) (help) - ↑ a b de Berg, Mark (2008). Computational Geometry: Algorithms and Applications (PDF). Springer-Verlag. ISBN 978-3-540-77973-5.
{{cite book}}
: Unknown parameter|coauthors=
ignored (|author=
suggested) (help) - ↑ Guibas, L. (1992). "Randomized incremental construction of Delaunay and Voronoi diagrams". Algorithmica. 7: 381–413. doi:10.1007/BF01758770.
{{cite journal}}
: Unknown parameter|coauthors=
ignored (|author=
suggested) (help) - ↑ Edelsbrunner, Herbert (1996). "Incremental Topological Flipping Works for Regular Triangulations". Algorithmica. 15: 223–241. doi:10.1007/BF01975867.
{{cite journal}}
: Unknown parameter|coauthors=
ignored (|author=
suggested) (help) - ↑ Computing Constrained Delaunay Triangulations
- ↑ G. Leach: Improving Worst-Case Optimal Delaunay Triangulation Algorithms. June 1992
- ↑ Cignoni, P. (1998). "DeWall: A fast divide and conquer Delaunay triangulation algorithm in Ed". Computer-Aided Design. 30 (5): 333–341. doi:10.1016/S0010-4485(97)00082-1.
{{cite journal}}
: Unknown parameter|coauthors=
ignored (|author=
suggested) (help) - ↑ A Comparison of Sequential Delaunay Triangulation Algorithms http://www.cs.berkeley.edu/~jrs/meshpapers/SuDrysdale.pdf
- ↑ http://www.cs.cmu.edu/~quake/tripaper/triangle2.html
- ↑ S-hull
- ↑ http://www.s-hull.org/
Credits
edit- This page was originally created from The Delaunay Triangulation at Wikipedia.